
I do this to vent because it was very jarring. I also received some negative comments and I wanted to take some time to address them directly. I learned some valuable lessons, so I wanted to summarize them. This semester we were back to teaching in person! I taught Calc 1, Calc 2, and Elementary Stats. Since we are only dealing with two dimensional data, this does not leave us many options, we use the vector : We want the vector to still maximize variance, while at the same time being orthogonal to. What should our other basis vector be? Since we still want to have a basis it makes sense to use an orthogonal unit vector obeying the same kinds of properties as the first vector. This means we need a second basis vector with which to express the data besides the “best” one from before. Suppose now that we do not want to reduce dimension, and instead we want to look at our data in two dimensions, but from a different perspective. This viewpoint is the one we take when extending the ideas into higher dimensions. The amount of spread for a given set of numbers can be measured in several ways, but we use “variance.” Thus, by dotting our data with the unit vector pointing in the direction of the best fit line, the transformed data has maximal variance. If we used any other unit vector besides the one pointing in the direction of the best fit line, the resulting numbers would be less spread out. The transformed data points are now just numbers living in one dimension, and they are all quite spaced apart! In fact, they are as spaced out as possible in the following sense. This reveals the dual way of thinking about what this transformation of the data does. Since we are thinking about two dimensional data this means the data can be written as a matrix with two columns: To this end let’s just think about two dimensional data, and let’s think about how we could reduce it to one dimension in a smart way.
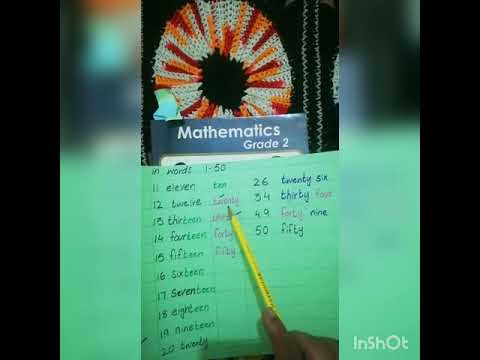
However, it took me a while to understand why it was a good way to reduce dimension, so that’s what I want to explain.

When you google “Principal Component Analysis” or “PCA” you find that it’s a pretty simple way of reducing the dimension of a matrix. Of course, there are many ways to make a bigger matrix smaller, but we want to do it in a useful way! Dimension reduction involves taking a large set of data represented as a matrix with many columns, and transforming it into a matrix with fewer columns. With this mini example the term “dimension reduction” may seem obvious. To summarize: we can either say there are 5 columns, 5 dimensions, or 5 features to this data set, and that there are 2 data points or 2 rows. Oddly, the number of features is also called the dimension of the data set, even though dimension of a matrix already means the number of rows times the number of columns. Meanwhile there are 5 “features” that encompass each data point, but really this is the number of columns. There are two data points and in general the number of rows of the matrix is the number of data points. For example, we might ask 2 people (things), for 5 pieces of information:Įxcuse the crude paint created pictures, but I’ve found this to be much faster than anything else! One very efficient way to do this is to use matrices. pieces of information, about a bunch of things, all at once. We want to consider a bunch of data, i.e. Data can really be any piece of information about something. The first thing I learned is that data scientists, just like statisticians, seem to use unnecessary language so I will do my best to write what math people might say instead of what a data scientist would say.ĭata is sought after and vacuumed up at alarming rates nowadays. To finalize my understanding of what we did I want to try to explain it here. I was excited to do this because I’ve always wanted to learn a little about data science, but also data science is especially good to know for a mathematician who does not have a tenure track position. I think this could be added to a linear algebra class for a nice real life application.
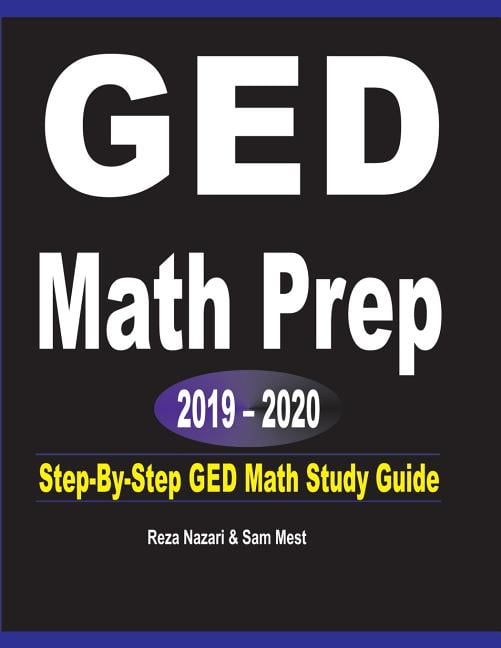
This Fall I had the opportunity to advise a student’s senior project! She wanted to study “dimension reduction” which, broadly speaking, consists of taking a large set of data and making it smaller, in various clever ways.
